Bayesian Analysis Simplified!
- Mazen Kherallah
- Nov 6, 2023
- 3 min read
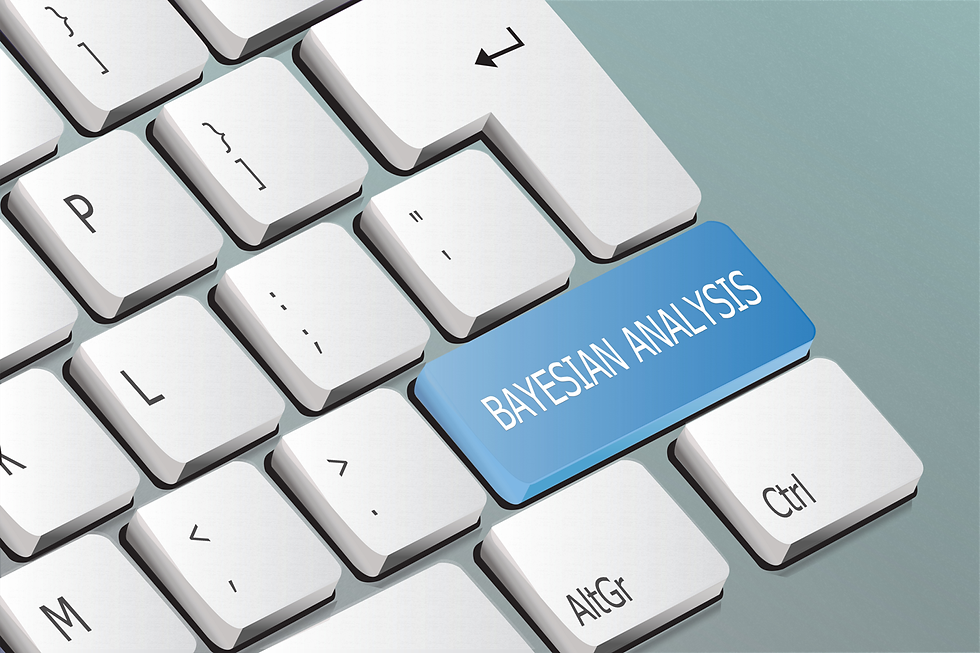
In the realm of clinical decision-making and medical research, Bayesian analysis has emerged as an indispensable statistical tool that merits a closer examination. This method offers a robust framework for integrating prior knowledge with emerging evidence, a process particularly relevant to the ever-evolving landscape of medical literature.
Here's a simplified explanation:
Picture a bag of mixed candies—some are red, and some are blue. If I asked you to guess the chances of picking a red one without peeking inside the bag, you might say it's 50/50. That's your prior probability—an initial guess based on what you know before you gather more information. This guess is based on the frequentist approach, which would rely solely on the long-term frequency of pulling red candies from similar bag.
Now, what if someone sneaks a peek and whispers to you that there are actually more blue candies than red? That little tidbit is your new evidence. In the medical world, this could be akin to fresh lab results or the latest findings from a clinical trial.
With this new piece of information, you adjust your initial guess. Maybe now you think there's a 70% chance you'll pull out a blue candy. Congratulations! You've just calculated a posterior probability. This is Bayesian analysis in a nutshell—adjusting your beliefs with new evidence.
In Bayesian analysis, the goal is to estimate the probability distribution of an unknown parameter based on observed data and prior knowledge or assumptions about the parameter. The prior distribution represents the initial belief or uncertainty about the parameter before observing the data, while the likelihood function represents the probability of observing the data given the parameter. The posterior distribution represents the updated belief or uncertainty about the parameter after observing the data and is proportional to the product of the prior distribution and the likelihood function.
Let's translate this to a medical scenario. A doctor might initially think there's a certain probability that a patient have a particular disease, based on symptoms and medical history. New test results come in, and they update that probability. It's like using each piece of evidence to get closer to the truth.
In clinical trials, researchers might start off with a neutral stance on a new drug. But as data from trial participants comes in showing improvement, they adjust their beliefs about the drug's effectiveness. Each new data point is a step toward a more informed conclusion.
Imagine combining all the whispers about what's in the candy bag from a bunch of people who have peeked inside. In medicine, this is like a meta-analysis—pooling data from several studies to get a clearer picture of how effective a treatment is.
Do you now have a better understanding of the basic concept of Bayesian Analysis?
Yes
Somewhat
Not a all!
The Takeaway
Bayesian analysis in medical literature allows for a more nuanced and dynamic approach to understanding clinical data. It starts with a prior probability, which is an initial estimate of an outcome based on existing knowledge. As new data becomes available from research or patient outcomes, this probability is updated, leading to a posterior probability that more accurately reflects the likelihood of a hypothesis being true in light of the new evidence.
This method is especially useful in clinical trials and diagnostics, where ongoing data can refine the understanding of a drug's effectiveness or a diagnostic test's accuracy. Bayesian analysis also aids in meta-analysis, where it can reconcile data from diverse studies to provide a comprehensive view of research findings. Overall, Bayesian analysis offers a flexible and iterative approach to data analysis and decision-making in healthcare, one that integrates prior knowledge with current evidence to come closer to the truth.
Comments